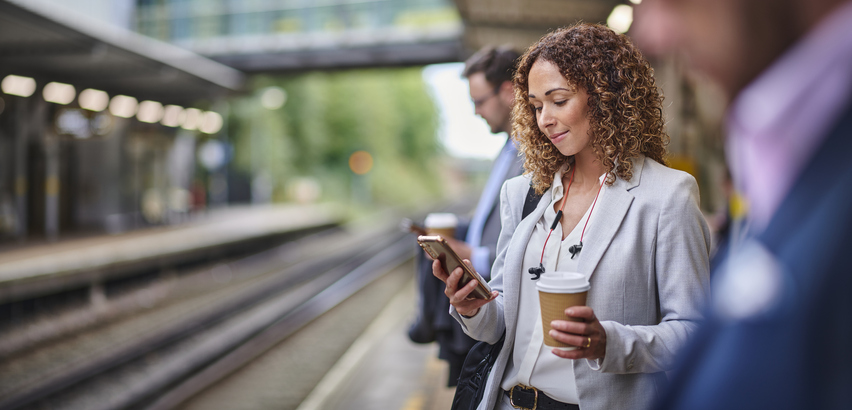
Article
The future of careers in data science
23 Sept, 20215mins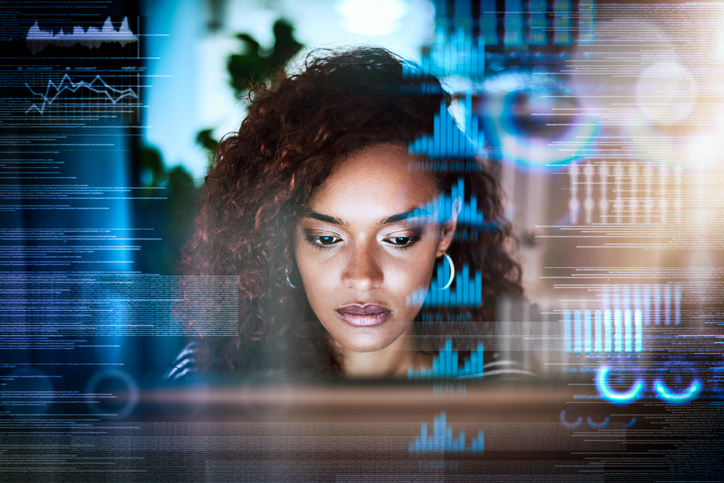
The nature of data science is changing. More aspects of the role are becoming automated, and this shift opens up data science to a wider audience. What do these changes mean for the field, and what will future data science careers look like?
Automation
Traditionally, data scientists have needed extensive programming knowledge from years of experience and, in most cases, a university degree in computer science or a related field. You would be hard-pushed to find a current job listing that doesn’t mention something like Python, Java or R as an essential requirement — because that’s what a data scientist is, someone who writes code and constructs complicated neural networks by hand — right?
Not quite, because automation has already changed the nature of the job. Automated tools mean the average data scientist spends less time on mundane tasks such as data preparation, data cleaning and writing code from scratch. Now automation is becoming incredibly sophisticated. For example, Google’s AutoML allows customers to train machine learning models and use them to gain insights from their own data. It is an extremely user-friendly platform requiring minimal data science knowledge.
A changing role
So, will data scientists soon be replaced by super-efficient automated tools? This seems unlikely. It is notoriously difficult to replicate the human element of data science. Automated tools are efficient but prone to error — particularly when models make their own assumptions — and lack the business knowledge to return relevant insights. Automation should make data science more productive and allow those in this area to focus on higher-value projects. This could also mean ‘higher-level’ data scientists specialise in narrower areas. So instead of being a data scientist, you might be an NLP expert or a recommendations scientist.
Wider access
While qualified data scientists will still be in demand for higher-level projects, automation will make the field more accessible than ever. Companies without a budget for a dedicated data science team can utilise automated tools to understand their business better. Future data science roles could become two-tier, with non-data science jobs requiring some experience with automated tools and specialist data scientist jobs specifically geared towards providing those top-level insights. Regardless, automation should increase demand for data scientists rather than decrease it by making the field accessible to the general population.
New roles
Not only will automation change the nature of existing roles, but it could create new jobs, particularly to help solve the additional problems that automation will create. For example, most data scientists use a wide range of open-source tools, such as Python and R, which contain hundreds of add-on packages. This results in what a recent Forbes article referred to as a ‘wild west’ collection of systems and tools, which increases both operational and security risks to a business. Therefore, with increased reliance on automated tools, there will be a greater need for organisational roles, such as Chief Information Officers (CIOs), to help mitigate this risk. This will be similarly important for businesses utilising data science tools for the first time (as they may lack the knowledge to foresee operational issues, such as a tool going out of date) and those with large data science teams (who are likely to rely on numerous tools).
The future of data science is bright. As mentioned by Techcrunch, we have already seen what happens to a field that becomes more accessible — increased automation in software engineering has only increased demand for programmers. The nature of the role might change, but we ultimately live in a data-driven world, and the need for talented individuals in this area is likely to grow for many years to come.
If you are a Data Science professional or your company wants to grow its Data Science teams, contact McGregor Boyall today and find out how our expert recruiters can help you.